Explainable AI in Asset Management
When using AI in asset management, the significance of explainability and reliability cannot be overstated. These aspects go beyond mere features; they form fundamental principles that instill trust, ensure comprehensiveness, and contribute to the facilitation of effective decision-making.
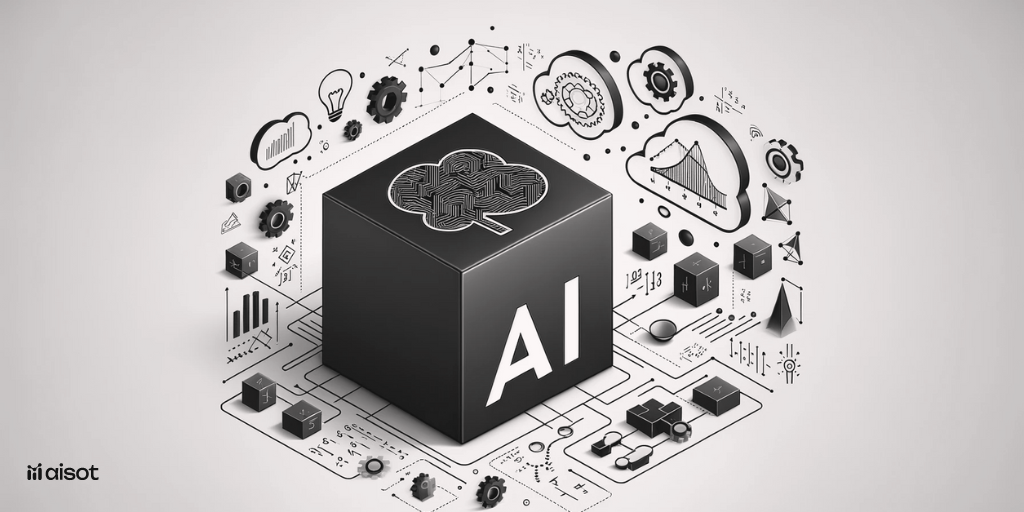
The term “explainable AI” describes AI models, their anticipated impacts, and potential biases. It plays a crucial role in characterizing the accuracy, fairness, transparency, and outcomes of AI-powered decision-making processes. Adopting explainable AI practices is essential for fostering a responsible approach to AI development. As AI continues to advance, there is an increasing challenge for humans to comprehend and trace the decision-making process of algorithms. This transformation often turns the entire calculation process into what is commonly known as a "black box," rendering it inscrutable. These black box models, generated directly from data, can be so complex that even their creators—engineers or data scientists—might struggle to understand or explain the internal workings, leading to a lack of transparency in how AI algorithms arrive at specific results.
In the intricate landscape of investment decisions, the application of AI has become a game-changer, promising unparalleled insights and efficiencies. However, the perceived black-box nature of many AI systems often raises concerns about the opacity of decision-making processes. In this exploration, we delve into the practical application of explainable AI for investments, unraveling how it adds a layer of clarity to the complex world of financial decision-making.
In asset management, two pivotal pillars uphold the integrity and effectiveness of explainable AI: 'relevant data' and 'understandable architecture.' The significance of relevant data lies in its capacity to provide a clear and accurate representation of the underlying factors influencing investment decisions. By ensuring that the data fed into the AI algorithms is pertinent to the context and objectives, asset managers can enhance the transparency of their decision-making processes. Simultaneously, an understandable architecture is indispensable for demystifying the complex workings of AI models. An architecture that is comprehensible to stakeholders facilitates trust and fosters a deeper understanding of how AI-driven insights are derived. Together, these pillars not only bolster the explainability of AI in asset management but also instill confidence among investors, enabling more informed and accountable decision-making.
aisot's data sources
aisot uses a comprehensive approach to market analysis by incorporating various data sources. This includes market data, alternative data, and macroeconomic data. By amalgamating information from these diverse sources, aisot aims to enhance the accuracy and effectiveness of its predictions and insights. The incorporation of market data provides real-time information on price movements and trading activities, alternative data (e.g. from news) introduces unique perspectives, and macroeconomic data contributes to a broader understanding of economic interlinkages and trends.
Market Data
Market data encompasses a comprehensive range of financial information crucial for making informed investment decisions. This data spans real-time quotes, historical price movements, trading volumes, bid-ask spreads, and various other metrics that reflect the dynamic nature of financial markets. Reliable and relevant market data serves as the cornerstone for aisot’s AI Insights Engine. Access to accurate and timely market data is paramount for navigating the complexities of asset management and optimizing investment performance.
Alternative Data
Alternative data refers to non-traditional and unconventional sources of information, enabling the AI Insights Platform to generate insights into market trends, potential risks, and investment opportunities. Unlike traditional data sources such as financial statements and economic indicators, alternative data can include a wide range of unstructured information. This might encompass social media sentiment analysis, blog posts, satellite imagery, geolocation data, and other non-traditional datasets. By incorporating alternative data, aisot aims to uncover unique perspectives and gain a competitive edge in understanding market dynamics.
Macroeconomic Data
Macroeconomic data includes a broad set of indicators and statistics, provide a high-level overview of a country's or region's overall economic health and performance. These indicators play a crucial role in shaping investment strategies and decisions. Key macroeconomic data includes measures such as Gross Domestic Product (GDP), inflation rates, unemployment figures, interest rates, and government fiscal policies. Understanding macroeconomic data is essential for assessing economic regimes (prevailing conditions in the broader economy, such as periods of growth, recession, or stability).
The architecture of the AI Insights Platform is intricately crafted with this principle at its core. Each element within the AI Insights Platform is designed to be traceable and explainable, ensuring that the rationale behind every decision driven by AI is readily accessible. This transparency in architecture serves multiple objectives. It enables our clients to grasp the underlying logic behind investment insights, guaranteeing that each provided insight aligns seamlessly with their investment objectives. Additionally, it fosters trust in AI-driven decisions, allowing one to follow the trajectory from data to recommendation and instilling confidence in the decision-making process.
aisot’s proprietary AI technology
aisot's AI for asset management uses a proprietary AI methodology that seamlessly integrates different forms of machine learning, natural language processing, and quant finance. In the following are selected concepts that are integrated into the AI Insights Platform.
Financial time series forecasting with Long Short-Term Memory (LSTM)
Financial time-series forecasting with Long Short-Term Memory (LSTM) networks involves utilizing the capabilities of LSTM, a type of recurrent neural network, to predict future values of financial metrics based on historical data. LSTM excels in handling sequential dependencies in time-series data, capturing long-term trends, and learning complex relationships. Its memory cells, adaptability to various time horizons, and ability to automatically learn relevant features make it well-suited for financial forecasting tasks. By leveraging LSTM's strengths, practitioners can enhance their models' accuracy and predictive power, particularly in capturing non-linear patterns and adapting to changing market conditions within the dynamic landscape of financial markets. Learn more: Guo, Tian, Tao Lin, and Nino Antulov-Fantulin. "Exploring interpretable LSTM neural networks over multi-variable data." International conference on machine learning. PMLR, 2019.
Volatility and Risk Analysis
aisot applies a sophisticated portfolio optimization approach to achieve a superior balance of expected returns and portfolio volatility. The platform enables usage of various metrics, e.g., minimization of the total portfolio volatility, or maximization of the portfolio’s expected return for a given target volatility, while obeying a multitude of customizable real-world constraints. At the heart of the optimization process is the inclusion of AI-generated views on the performance and covariance of the instruments in the considered investment universe. This data-driven and adaptive approach, enhances the precision of volatility predictions and allows for real-time adjustments, aligning investment strategies with the dynamic nature of financial markets.
Sentiment Analysis (NLP)
aisot analyzes a constant stream of news and social media data using various Natural Language Models, including Large Language Models. The utilization of these advanced models allows aisot to comprehensively understand and process the intricate nuances embedded within textual data. Beyond mere comprehension, aisot takes a step further by quantifying the propagation and absorption dynamics of large-scale publicly available news articles sourced from the Internet, particularly those pertinent to financial markets. This entails assessing not only the content of the news but also the sentiment expressed, allowing for a nuanced understanding of market perceptions. This continuous and sophisticated analysis enables aisot’s AI Insights Platform to adapt to evolving market conditions, empowering asset managers with a more responsive and informed decision-making process.
Next Generation Neural Networks
aisot researchers have advanced neural networks by integrating principles from control theory, graph theory, and differential equations. This approach enhances the adaptability of neural networks to changing market conditions, improves the representation of interconnected financial instruments through graph modeling, and allows for a more sophisticated understanding of temporal dynamics using differential equations. This integrated approach provides asset managers with a more powerful toolkit for analyzing and forecasting market behavior, enhancing the precision and adaptability of AI-driven strategies in the dynamic landscape of financial markets.
In the evolving investment landscape, integrating cutting-edge AI platforms like the AI Insights Platform is more than an option; it's a vital strategic imperative for contemporary asset management. The utilization of the AI Insights Platform empowers investment professionals with a suite of indispensable tools. Through this platform, they gain seamless access to portfolio optimization capabilities, unlocking a new echelon of customization. Furthermore, the platform facilitates advanced analysis, allowing for a more profound understanding of market trends and risk factors. One of its standout features is enhanced explainability, enabling investment professionals to decipher and articulate the rationale behind AI-driven decisions. As a result, the AI Insights Platform becomes an indispensable ally, equipping asset managers with the means to navigate complexities and make well-informed decisions in the ever-evolving financial landscape.